Python NumPy: A Comprehensive Guide
Updated on : 12 December, 2024, 10:00 IST
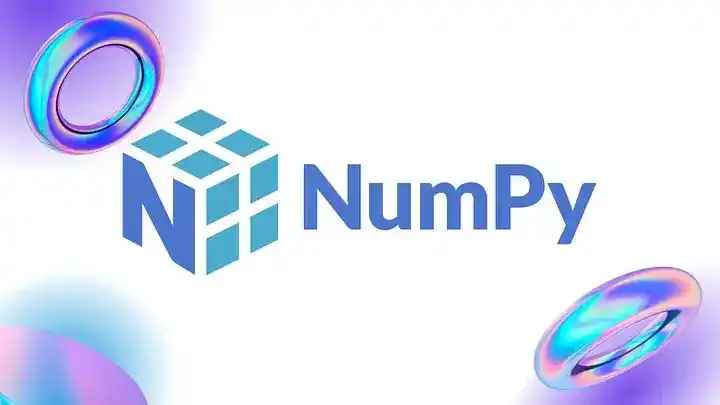
Image Source: freepik.com
Table Of Contents
- 1. What is NumPy?
- 2. Why Use NumPy?
- 3. Getting Started with NumPy
- 4. Creating Arrays
- 5. Array Attributes
- 6. Reshaping Arrays
- 7. Indexing and Slicing
- 8. Advanced Operations
- 9. Mathematical Operations
- 10. Broadcasting Example
- 11. Statistical Functions
- 12. Random Number Generation
- 13. Applications of NumPy
- 14. Data Visualization with NumPy
- 15. Conclusion
Table Of Contents
What is NumPy?
NumPy, short for Numerical Python, is an essential library in the Python programming ecosystem, particularly for scientific computing and data analysis. It provides support for large, multi-dimensional arrays and matrices, along with a collection of mathematical functions to operate on these arrays. This blog post will delve into what NumPy is, its features, benefits, and practical applications, providing a comprehensive guide for beginners and experienced users alike.
Key Features of NumPy
Feature | Description |
---|---|
N-dimensional Arrays | The core feature of NumPy is its powerful N-dimensional array object (ndarray ). This allows users to create arrays of any dimension—1D (vectors), 2D (matrices), or even higher dimensions. |
Performance | NumPy arrays are significantly faster than Python lists due to their compact memory layout. Operations on NumPy arrays can be up to 50 times faster than equivalent operations on lists. |
Broadcasting | This feature allows NumPy to perform arithmetic operations on arrays of different shapes without the need for explicit replication of data. |
Mathematical Functions | NumPy comes with a plethora of built-in functions for mathematical operations such as linear algebra, statistical analysis, and Fourier transforms. |
Integration with Other Libraries | NumPy serves as the foundation for many other libraries in the Python ecosystem, including Pandas for data manipulation and Matplotlib for data visualization. |
Why Use NumPy?
The advantages of using NumPy over traditional Python lists are numerous:
-
Speed and Efficiency: NumPy's array operations are executed at C speed due to its implementation in C and C++. This results in faster execution times for numerical computations.
-
Memory Efficiency: A NumPy array uses less memory than a Python list containing the same data. This efficiency is crucial when dealing with large datasets common in data science applications.
-
Ease of Use: The syntax for creating and manipulating arrays is straightforward. Functions like
np.array()
,np.zeros()
, andnp.ones()
make it easy to generate arrays quickly. -
Rich Functionality: With functions for reshaping arrays, performing mathematical operations, and generating random numbers, NumPy simplifies many tasks that would otherwise require complex coding.
Getting Started with NumPy
To begin using NumPy, you first need to install it if it isn't already installed. You can do this using pip:
pip install numpy
Once installed, you can import it into your Python script:
import numpy as np
Creating Arrays
Creating a NumPy array can be done using the np.array()
function. Here are some examples:
# Creating a 1D Array
arr_1d = np.array([1, 2, 3])
print("1D Array:", arr_1d) # Output: [1 2 3]
# Creating a 2D Array (Matrix)
arr_2d = np.array([[1, 2], [3, 4]])
print("2D Array:\n", arr_2d)
# Output:
# [[1 2]
# [3 4]]
# Creating a 3D Array
arr_3d = np.array([[[1], [2]], [[3], ]])
print("3D Array:\n", arr_3d)
# Output:
# [[[1]
# [2]]
#
# [[3]
# ]]
In these examples:
- A 1D array is created to hold a sequence of numbers.
- A 2D array (matrix) is constructed using nested lists.
- A 3D array is formed by nesting lists further.
Array Attributes
NumPy arrays have several useful attributes:
- Shape: The shape of an array can be accessed using the
.shape
attribute.
print("Shape of 2D Array:", arr_2d.shape) # Output: (2, 2)
This attribute returns a tuple representing the dimensions of the array.
- Data Type: The data type of the elements in an array can be checked with the
.dtype
attribute.
print("Data Type:", arr_1d.dtype) # Output: int64
This shows the type of elements stored in the array.
Reshaping Arrays
You can reshape an array without changing its data using the .reshape()
method:
reshaped_arr = arr_1d.reshape((3, 1))
print("Reshaped Array:\n", reshaped_arr)
This code changes the shape of arr_1d
from a one-dimensional array to a two-dimensional column vector.
Indexing and Slicing
NumPy supports advanced indexing and slicing techniques:
Basic Indexing:
print("First Element:", arr_1d) # Output: 1
print("Second Row:", arr_2d[1]) # Output: [3,4]
Here we access specific elements from the arrays directly by their indices.
Slicing:
print("Slice from index 1 to end:", arr_1d[1:]) # Output: [2,3]
This retrieves a subset of the array from index 1
to the end.
Multi-dimensional Slicing:
print("First Column of 2D Array:", arr_2d[:,0]) # Output: [1,3]
This selects all rows from the first column of a two-dimensional array.
Advanced Operations
Mathematical Operations
NumPy allows you to perform element-wise operations easily:
a = np.array([1,2,3])
b = np.array([4,5,6])
print("Element-wise Addition:", a + b) # Output: [5,7,9]
print("Element-wise Multiplication:", a * b) # Output: [4,10,18]
These operations demonstrate how you can perform arithmetic directly on arrays.
Broadcasting Example
Broadcasting enables operations between arrays of different shapes:
c = np.array([[1], [2], [3]])
d = np.array([4,5])
result = c + d # Broadcasting happens here
print("Broadcasting Result:\n", result)
In this example:
- The smaller array
c
is broadcasted acrossd
allowing element-wise addition despite differing shapes.
Statistical Functions
NumPy provides numerous statistical functions that can be applied directly to arrays:
data = np.array([10,20,30])
print("Mean:", np.mean(data)) # Output: Mean value (20.0)
print("Standard Deviation:", np.std(data)) # Output: Std deviation value (10.0)
These functions facilitate quick statistical analysis on datasets.
Random Number Generation
NumPy also includes functionality for generating random numbers:
random_array = np.random.rand(5) # Generate an array with random floats between 0 and 1
print("Random Array:", random_array)
# Create a random integer array
random_int_array = np.random.randint(0, high=10, size=(3,4))
print("Random Integer Array:\n", random_int_array)
Here we generate random floats and integers which are useful in simulations or testing scenarios.
Applications of NumPy
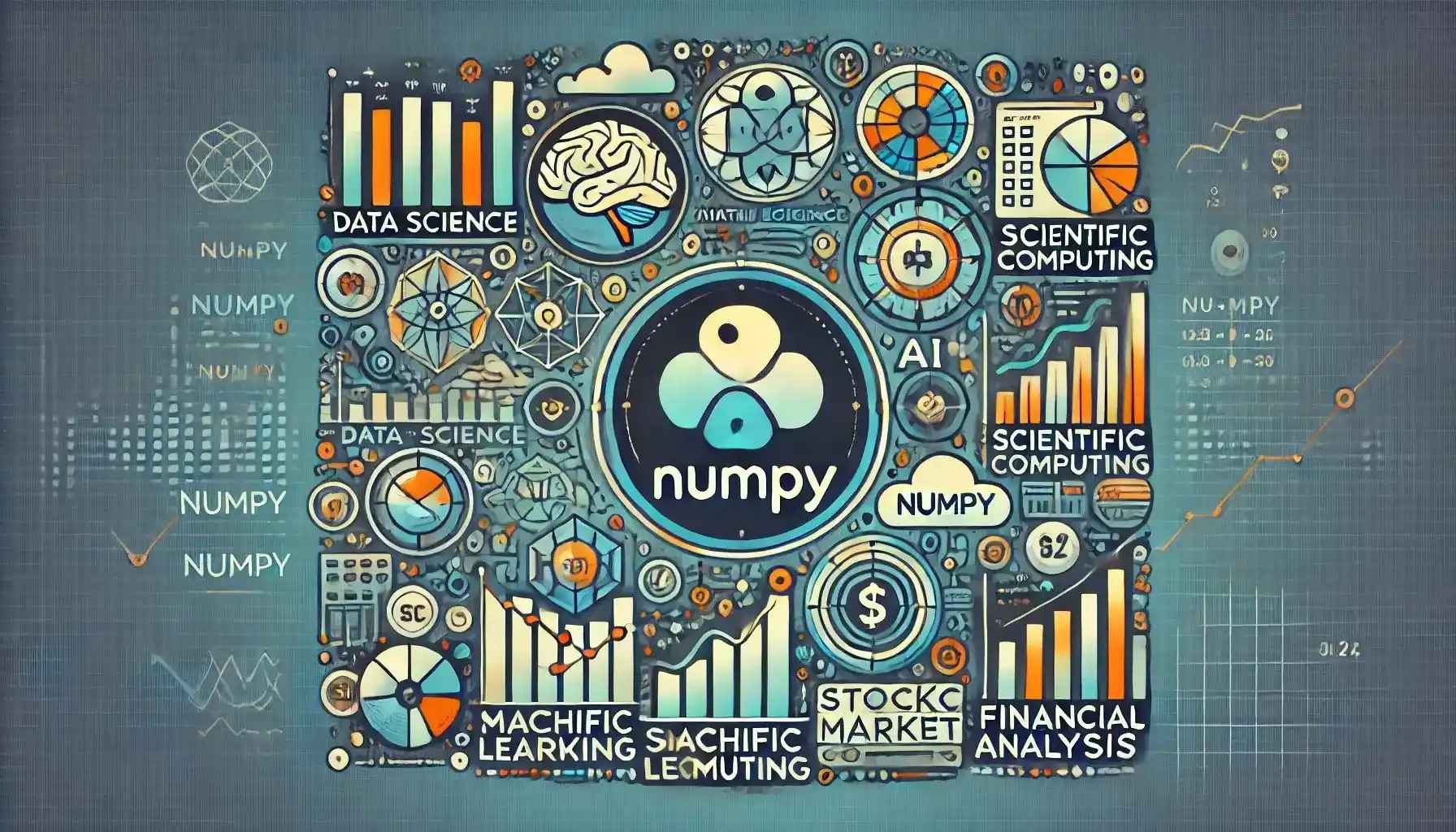
Image Source: AI-Generated
Given its efficiency and powerful capabilities, NumPy is widely used across various domains:
Domain | Description |
---|---|
Data Science | Data scientists use NumPy for data manipulation and analysis due to its speed and ease of use. |
Machine Learning | Libraries like TensorFlow and scikit-learn rely on NumPy for handling numerical data efficiently. |
Scientific Computing | Researchers utilize NumPy for simulations and calculations that require high performance. |
Financial Analysis | Analysts use NumPy for modeling financial scenarios due to its ability to handle large datasets effectively. |
Data Visualization with NumPy
While primarily known for numerical computations, NumPy also plays a crucial role in data visualization. It integrates seamlessly with libraries like Matplotlib and Seaborn to enable efficient plotting.
Integration with Visualization Libraries
- Matplotlib:
import matplotlib.pyplot as plt
x = np.linspace(0,10,100) # Generate values from 0 to 10
y = np.sin(x) # Calculate sine values
plt.plot(x,y)
plt.title("Sine Wave")
plt.xlabel("X-axis")
plt.ylabel("Y-axis")
plt.grid()
plt.show()
This code snippet generates a sine wave plot by calculating sine values over a range.
- Seaborn:
import seaborn as sns
data = np.random.rand(10,12) # Generate random data for heatmap
sns.heatmap(data)
plt.title("Random Heatmap")
plt.show()
Here we create a heatmap visualization from randomly generated data.
Conclusion
NumPy is a powerful library that forms the backbone of numerical computing in Python. Its ability to handle large datasets efficiently makes it indispensable in various fields such as data science and machine learning. By understanding its core features—like N-dimensional arrays, broadcasting capabilities, and rich mathematical functions—you can leverage NumPy's full potential in your projects.
Whether you're just starting out or looking to deepen your understanding of numerical computing in Python, mastering NumPy will significantly enhance your programming toolkit.