Neural Networks: The Backbone of AI and Machine Learning
Updated on : 25 November, 2024, 12:20 IST
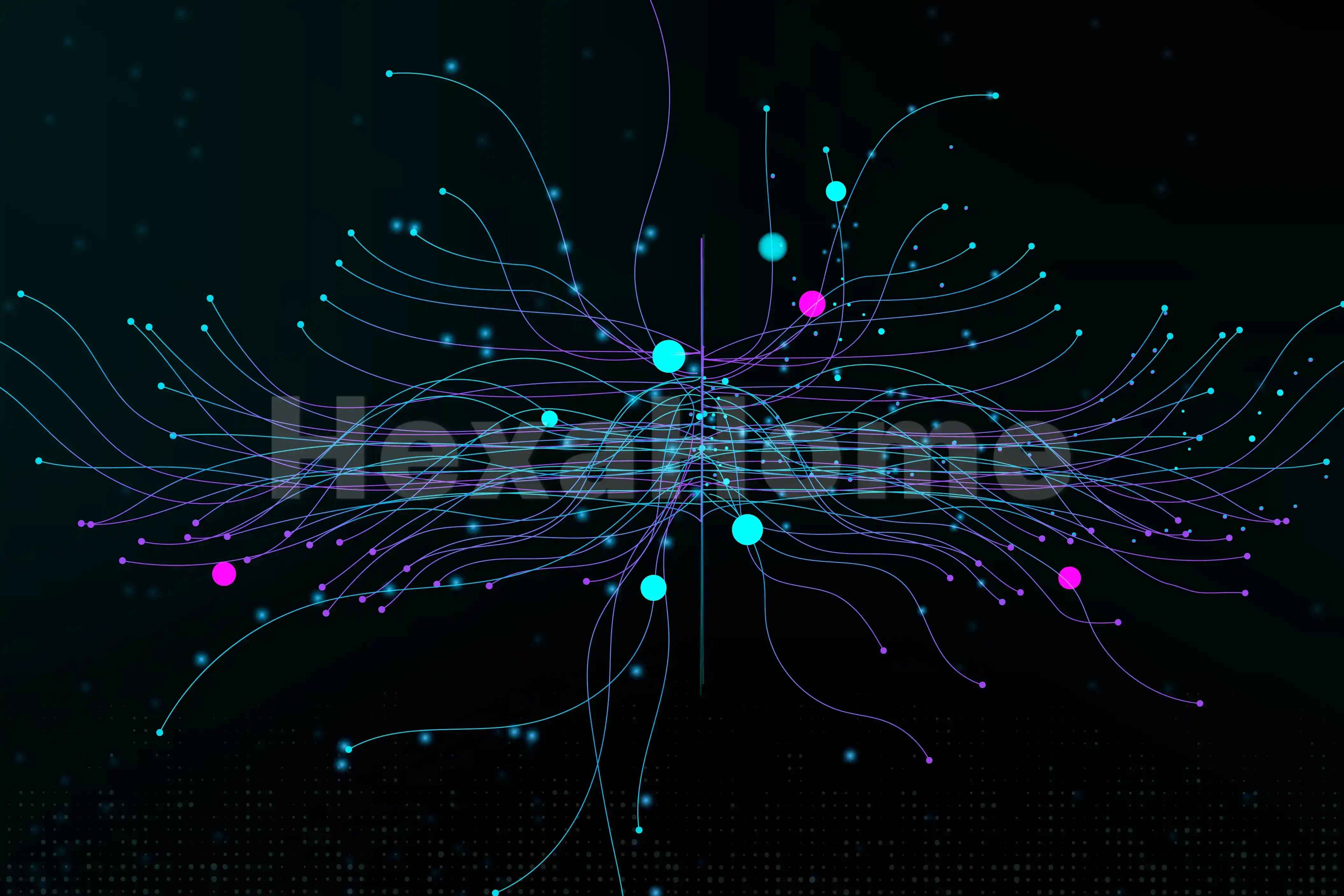
Image Source: Freepik.com
Table Of Contents
Neural networks have become a cornerstone of artificial intelligence (AI) and machine learning (ML), revolutionizing how we approach complex problems across various domains. From image recognition to natural language processing, neural networks are at the forefront of technological innovation. This blog will delve deep into the world of neural networks, exploring their architecture, types, training processes, applications, and future prospects.
What is a Neural Network?
At its core, a neural network is a computational model inspired by the way biological neural networks in the human brain operate. It consists of interconnected nodes, or artificial neurons, organized in layers.
These layers include:
- Input Layer: The first layer that receives input data.
- Hidden Layers: Intermediate layers where computations and transformations occur.
- Output Layer: The final layer that produces the output. Each connection between neurons has an associated weight, which adjusts as learning progresses, allowing the network to make predictions or classifications based on input data.
How Neural Networks Work?
Neural networks operate through a process called feedforward, where data is passed through the network from the input layer to the output layer. Each neuron processes its input by applying a weighted sum followed by an activation function, which determines whether the neuron should be activated or not.
- Activation Functions: Activation functions introduce non-linearity into the model, enabling it to learn complex patterns.
Common activation functions include:
- Sigmoid: Outputs values between 0 and 1, often used in binary classification.
- ReLU (Rectified Linear Unit): Outputs zero for negative inputs and the input itself for positive inputs, helping to mitigate the vanishing gradient problem.
- Softmax: Used in multi-class classification problems to produce probabilities for each class.
- Backpropagation: The training of neural networks relies heavily on a technique called backpropagation. This algorithm calculates the gradient of the loss function with respect to each weight by applying the chain rule of calculus. The goal is to minimize the loss function—an indicator of how well the network's predictions match actual outcomes—by adjusting weights accordingly.
Types of Neural Networks
Neural networks come in various architectures tailored for specific tasks.
Here are some of the most common types:
- Feedforward Neural Networks (FNN): The simplest type of neural network where connections between nodes do not form cycles. Information moves in one direction—from input to output—making it suitable for straightforward tasks like regression and classification.
- Convolutional Neural Networks (CNN): CNNs are specialized for processing structured array data such as images. They utilize convolutional layers that apply filters to capture spatial hierarchies in data, making them ideal for tasks like image recognition and object detection.
- Recurrent Neural Networks (RNN): RNNs are designed for sequential data processing, allowing information from previous inputs to influence current outputs. This architecture is particularly useful for time series analysis and natural language processing tasks like speech recognition and text generation.
- Generative Adversarial Networks (GAN): GANs consist of two neural networks—a generator and a discriminator—that compete against each other. The generator creates fake data while the discriminator evaluates its authenticity, leading to improved performance in generating realistic data such as images or audio.
Training Neural Networks
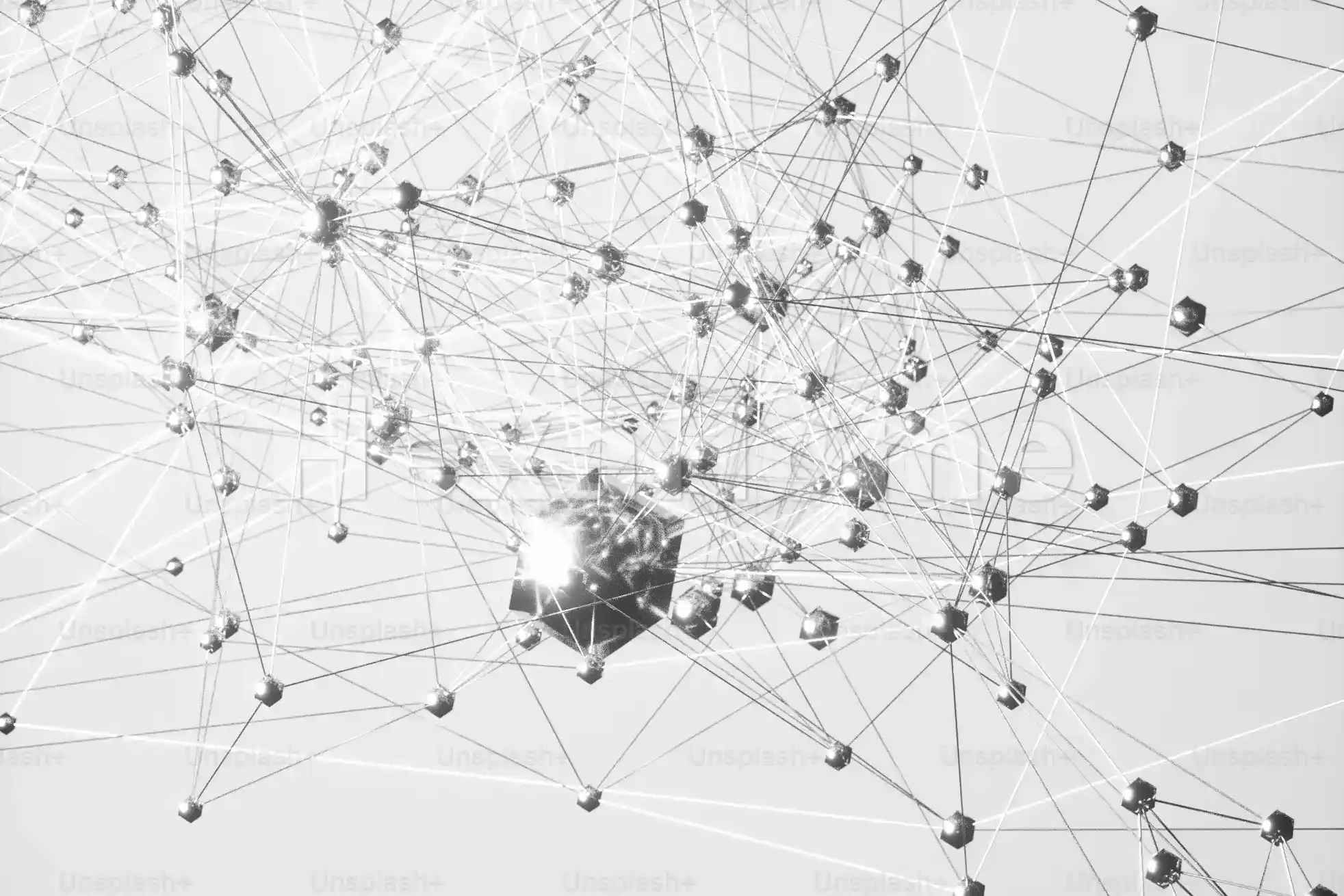
Image Source: Freepik.com
Training a neural network involves several key steps:
- Data Preparation: Quality data is crucial for effective training. Data must be preprocessed—normalized or standardized—to ensure that all features contribute equally to learning.
- Splitting Data: Typically, datasets are divided into three subsets:
- Training Set: Used to train the model.
- Validation Set: Used to tune hyperparameters and prevent overfitting.
- Test Set: Used to evaluate model performance on unseen data.
- Choosing Hyperparameters: Hyperparameters such as learning rate, batch size, and number of epochs significantly influence training outcomes. Techniques like grid search or random search can help identify optimal hyperparameter values.
- Optimization Algorithms: Various optimization algorithms can be employed during training:
- Stochastic Gradient Descent (SGD): Updates weights based on individual training examples.
- Adam: Combines advantages from both AdaGrad and RMSProp, adapting learning rates based on moment estimates.
Applications of Neural Networks
Neural networks have found applications across numerous fields:
- Image Recognition: CNNs excel in image classification tasks, enabling systems like facial recognition software and autonomous vehicles to interpret visual information accurately.
- Natural Language Processing: RNNs and transformer-based architectures power applications such as chatbots, language translation services, and sentiment analysis tools.
- Healthcare: Neural networks assist in diagnosing diseases through medical imaging analysis, predicting patient outcomes, and personalizing treatment plans based on genetic information.
- Finance: In finance, neural networks are used for credit scoring, fraud detection, algorithmic trading, and risk assessment by analyzing vast amounts of transaction data.
Challenges and Limitations
Despite their power, neural networks face several challenges:
- Overfitting: When a model learns too much from training data including noise—it may perform poorly on unseen data. Techniques such as dropout regularization or early stopping can help mitigate overfitting.
- Interpretability: Neural networks are often viewed as "black boxes," making it difficult to understand how they arrive at specific decisions or predictions. Research into explainable AI aims to address this issue by providing insights into model behavior.
- Computational Resources: Training deep neural networks requires significant computational power and memory resources, often necessitating specialized hardware like GPUs or TPUs.
The Future of Neural Networks
The future of cloud computing looks promising with emerging trends such as:
-
Edge Computing: Bringing computation closer to data sources to reduce latency.
-
Artificial Intelligence Integration: Enhancing cloud services with AI capabilities for better analytics and automation.
-
Increased Focus on Security: As cyber threats evolve, cloud providers are investing heavily in advanced security measures
Summing it Up
Neural networks have transformed how we approach complex problems across various domains by mimicking human cognitive processes through interconnected layers of artificial neurons. As advancements continue in this field driven by research and technological innovation the potential applications of neural networks will only expand further. Understanding neural networks is crucial for anyone looking to delve into AI and machine learning; they serve as foundational tools powering many modern technologies we rely on today. Whether you’re interested in developing intelligent systems or simply curious about how machines learn from data, grasping the principles behind neural networks will provide valuable insights into this fascinating domain of artificial intelligence. As we look ahead into an era where AI becomes increasingly integrated into our daily lives, embracing these technologies will be essential not just for developers but for society as a whole to harness their potential responsibly and ethically while addressing challenges along the way. whether you’re exploring applications in healthcare or developing cutting-edge image recognition systems, neural networks represent a critical component driving innovation across industries today and their journey has only just begun!