Natural Language Processing: Key Concepts and Applications
Updated on : 18 November, 2024, 10:00 IST
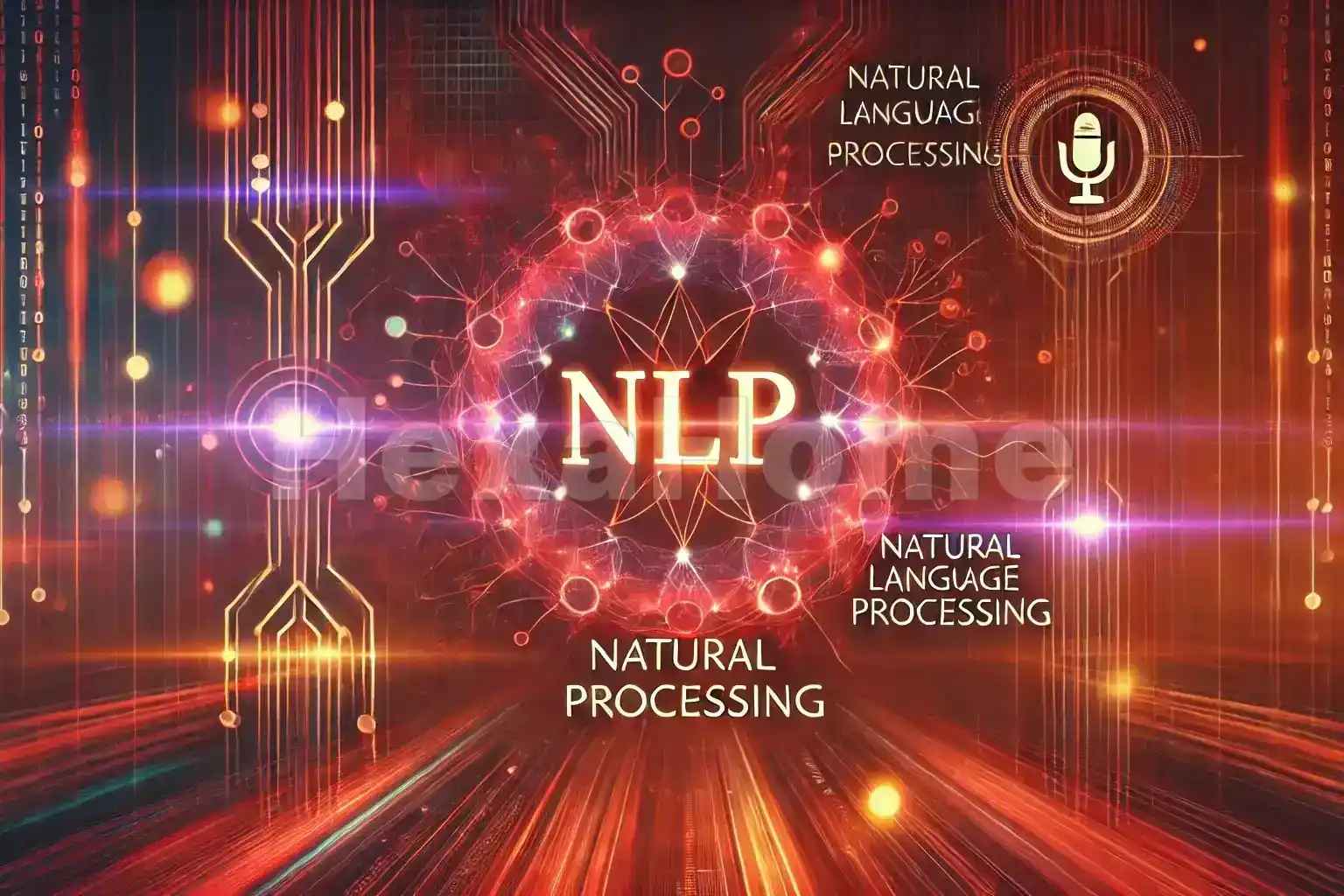
Image Source: AI-generated
Table Of Contents
- 1. What is Natural Language Processing?
- 2. The Evolution of Natural Language Processing
- 3. Key Components of Natural Language Processing
- 4. Applications of Natural Language Processing
- 5. Challenges in Natural Language Processing
- 6. Future Trends in Natural Language Processing
- 7. Conclusion
Table Of Contents
What is Natural Language Processing?
Natural Language Processing refers to the ability of computers to understand, interpret, and generate human language. It combines computational linguistics—rule-based modeling of human language—with machine learning, statistical methods, and deep learning to enable machines to process language data.
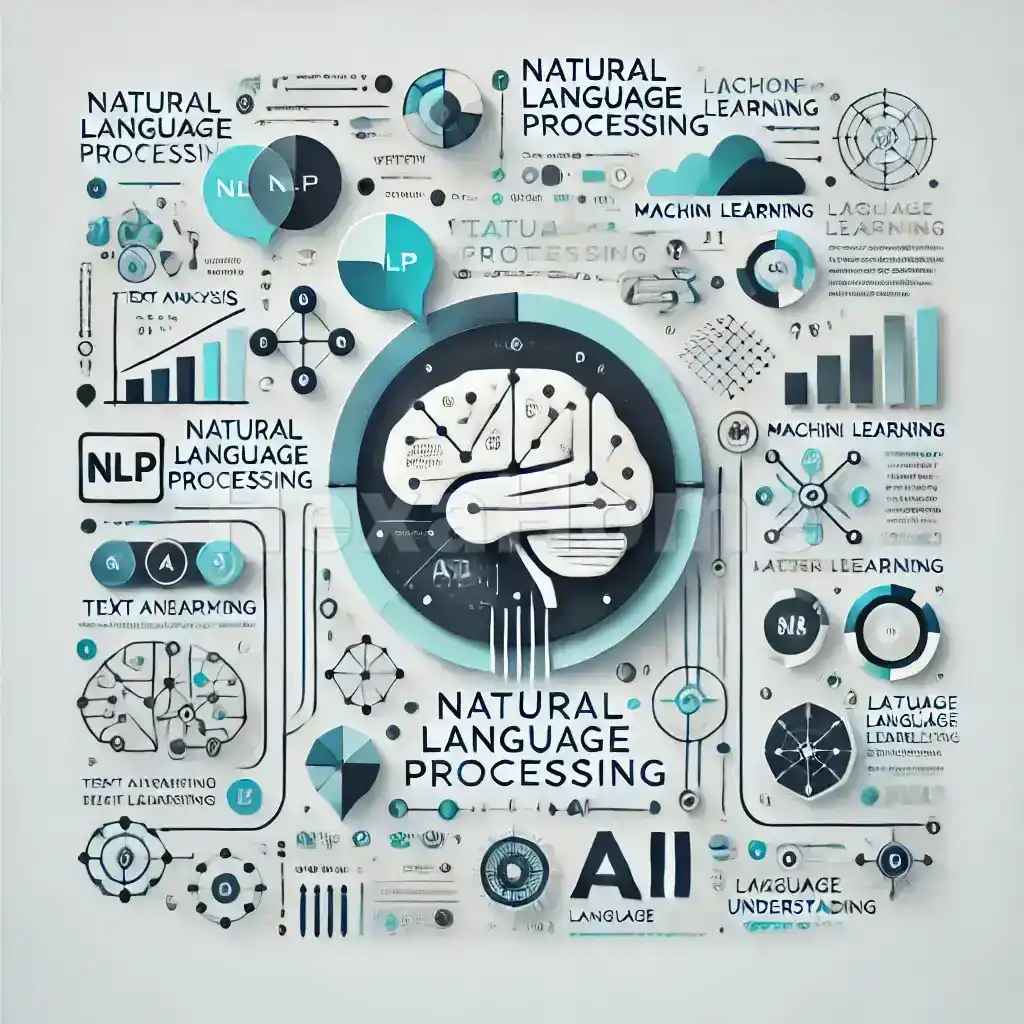
Image Source: AI-generated
NLP encompasses several tasks, including:
- Text Analysis: Understanding the structure and meaning of text.
- Speech Recognition: Converting spoken language into text.
- Sentiment Analysis: Determining the emotional tone behind a series of words.
- Machine Translation: Translating text from one language to another.
The ultimate goal of NLP is to facilitate seamless interaction between humans and machines by allowing computers to understand language as humans do.
The Evolution of Natural Language Processing
The journey of NLP began in the 1950s when researchers started exploring ways to enable machines to understand human language. Early attempts included rule-based systems that relied on predefined grammatical rules. However, these systems struggled with the complexities and nuances of natural language.
With advancements in machine learning and the availability of large datasets, NLP has evolved significantly. The introduction of deep learning techniques has further enhanced the capabilities of NLP systems. Today, models like OpenAI's GPT-3 and Google's BERT are capable of understanding context, generating coherent text, and even engaging in conversations that mimic human interaction.
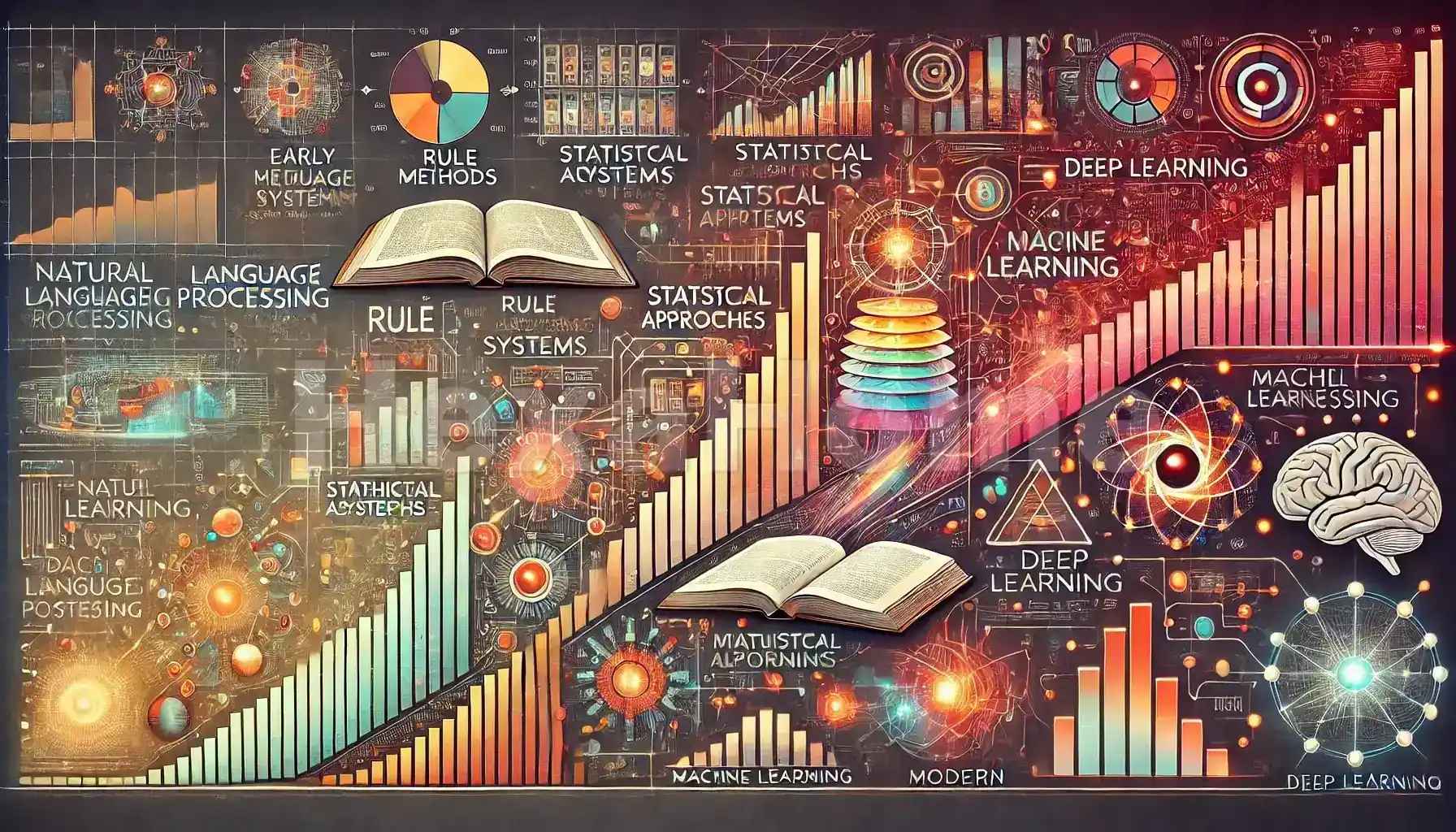
Image Source: AI-generated
Key Components of Natural Language Processing
To understand how NLP works, it's essential to explore its key components:
-
Tokenization: This process involves breaking down text into smaller units called tokens (words or phrases). Tokenization helps in analyzing the structure of sentences.
-
Part-of-Speech Tagging: This step assigns grammatical categories (nouns, verbs, adjectives) to each token. It helps in understanding the role each word plays in a sentence.
-
Named Entity Recognition (NER): NER identifies and classifies key elements in text into predefined categories such as names of people, organizations, locations, dates, etc.
-
Dependency Parsing: This technique analyzes the grammatical structure of a sentence to understand how words relate to each other.
-
Sentiment Analysis: Sentiment analysis determines the emotional tone behind words—whether they convey positive, negative, or neutral sentiments.
-
Machine Translation: This component focuses on translating text from one language to another while preserving its meaning.
Applications of Natural Language Processing
NLP has found applications across various industries due to its ability to process vast amounts of unstructured data efficiently. Here are some notable applications:
1. Chatbots and Virtual Assistants
Chatbots powered by NLP can engage users in natural conversations. They are used in customer service to answer queries, provide support, and assist with transactions. Virtual assistants like Siri, Alexa, and Google Assistant leverage NLP to understand voice commands and perform tasks such as setting reminders or playing music.
2. Sentiment Analysis
Businesses use sentiment analysis tools to gauge public opinion about their products or services by analyzing social media posts, reviews, and feedback. By understanding customer sentiment, companies can make informed decisions about marketing strategies and product improvements.
3. Machine Translation
Services like Google Translate utilize NLP algorithms to translate text between languages accurately. While early translation systems struggled with context and idiomatic expressions, modern NLP techniques have significantly improved translation quality.
4. Text Summarization
NLP can automatically summarize long articles or documents by extracting key points and presenting them concisely. This application is particularly useful for news aggregation services or research databases where users need quick insights without reading entire texts.
5. Speech Recognition
NLP plays a crucial role in converting spoken language into written text through speech recognition technologies. Applications range from voice-to-text transcription services to virtual assistants that respond to voice commands.
6. Email Filtering
Email providers use NLP algorithms to categorize incoming messages as spam or legitimate based on their content. By analyzing patterns in email data, these systems can effectively filter out unwanted messages before they reach users' inboxes.
Challenges in Natural Language Processing
Despite its advancements, NLP faces several challenges:
-
Ambiguity: Human language is inherently ambiguous; words can have multiple meanings depending on context (e.g., "bat" can refer to a flying mammal or a sports equipment). Resolving ambiguity remains a significant hurdle for NLP systems.
-
Sarcasm and Irony: Detecting sarcasm or irony poses challenges for machines as these expressions often convey meanings contrary to their literal interpretation.
-
Context Understanding: Understanding context is crucial for accurate interpretation; however, maintaining context over long conversations or texts can be complex for NLP models.
-
Data Privacy: As NLP systems often require vast amounts of data for training, concerns about data privacy and security arise—particularly when handling sensitive information.
-
Bias in Data: If training data contains biases (e.g., gender or racial biases), NLP models may inadvertently perpetuate these biases in their outputs.
Future Trends in Natural Language Processing
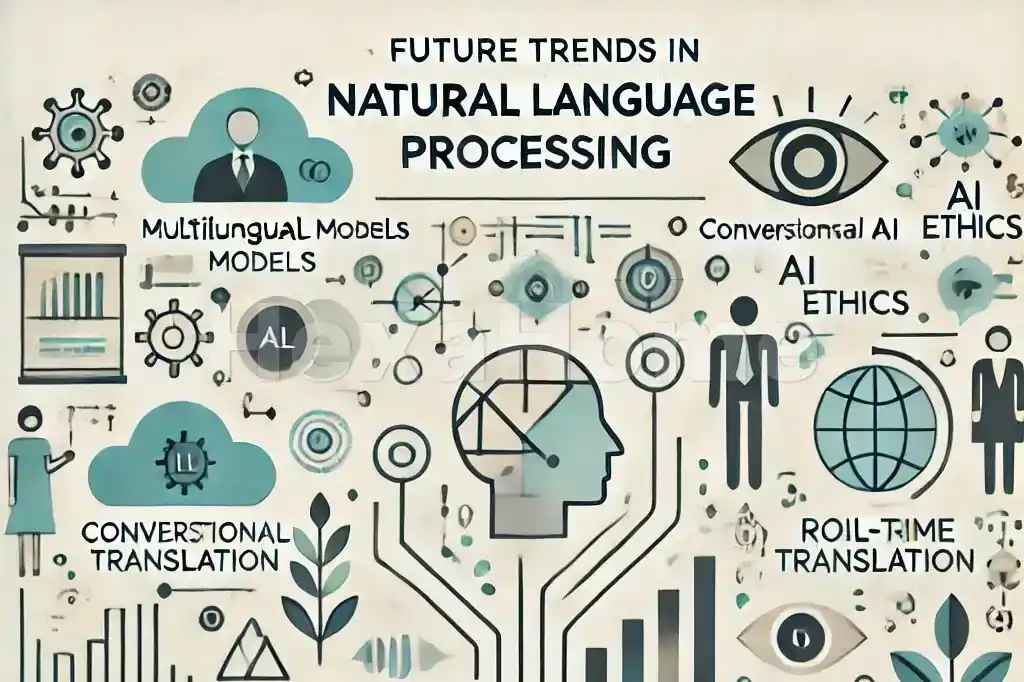
Image Source: AI-generated
As technology continues to evolve, several trends are shaping the future of NLP:
-
Improved Contextual Understanding: Ongoing research aims to enhance models' ability to maintain context over longer interactions and understand subtleties in human communication better.
-
Multimodal Learning: Combining textual data with other forms of input (e.g., images or audio) will enable more comprehensive understanding and interaction capabilities for AI systems.
-
Personalized Experiences: As NLP evolves, applications will become more personalized by leveraging user data and preferences to provide tailored responses and recommendations.
-
Ethical Considerations: The focus on ethical AI will grow as organizations strive to address issues related to bias, transparency, and accountability in NLP systems.
-
Integration with Other Technologies: NLP will increasingly integrate with other AI technologies such as computer vision and robotics to create more sophisticated applications capable of interacting with users across various platforms.
Conclusion
Natural Language Processing is revolutionizing how we interact with technology by enabling machines to understand human language more effectively than ever before. From chatbots that enhance customer service experiences to advanced translation tools that break down language barriers, the applications of NLP are vast and impactful.
While challenges remain—such as ambiguity in language and potential biases—ongoing advancements in machine learning and deep learning promise a bright future for this field. As we continue exploring the potential of NLP, it is clear that it will play an essential role in shaping our interactions with technology and enhancing our ability to communicate effectively across diverse platforms.
In summary, Natural Language Processing stands at the forefront of AI innovation—bridging the gap between human communication and machine understanding—and unlocking new opportunities for businesses and individuals alike as we navigate an increasingly digital world.
Additional Insights into Natural Language Processing
To further enrich your understanding of Natural Language Processing (NLP), let’s delve deeper into some specific techniques used within this domain:
Advanced Techniques Used in NLP
-
Stemming vs Lemmatization:
- Stemming reduces words to their root form by removing suffixes (e.g., "running" becomes "run"). However, it may not always produce a valid word.
- Lemmatization considers the context and converts words into their meaningful base form (e.g., "better" becomes "good"). It requires a deeper understanding of the word's meaning within its context.
-
Word Embeddings:
- Word embeddings are dense vector representations that capture semantic meanings based on word usage within large datasets (e.g., Word2Vec or GloVe). Words with similar meanings are located closer together in this vector space.
- These embeddings allow models to understand relationships between words beyond mere frequency counts used in traditional methods like Bag-of-Words (BoW).
-
Transformers:
- Transformers have revolutionized NLP by enabling models like BERT (Bidirectional Encoder Representations from Transformers) that consider context from both directions—left-to-right and right-to-left—when processing text.
- This architecture allows for better handling of long-range dependencies within sentences compared with previous sequential models like RNNs (Recurrent Neural Networks).
-
Attention Mechanisms:
- Attention mechanisms allow models to focus on specific parts of input sequences when generating outputs rather than treating all parts equally.
- This capability enhances performance on tasks such as translation where certain words may carry more significance than others depending on their position within sentences.
-
Dialogue Systems:
- Dialogue systems aim at creating conversational agents capable of engaging users naturally through back-and-forth interactions.
- These systems employ techniques from both NLU (Natural Language Understanding) for interpreting user inputs and NLG (Natural Language Generation) for crafting appropriate responses based on conversation history.
By understanding these advanced techniques within Natural Language Processing (NLP), you can appreciate how they contribute significantly towards enhancing machine comprehension capabilities while addressing challenges associated with human languages' complexity.
Final Thoughts
As we look towards the future of Natural Language Processing (NLP), it’s evident that its impact will only grow stronger across various sectors—from healthcare utilizing chatbots for patient engagement—to finance employing sentiment analysis tools for market predictions—the possibilities are endless!
Investing time into learning about this fascinating field not only equips individuals with valuable skills but also empowers organizations aiming at leveraging AI technologies effectively while fostering innovation through improved communication interfaces between humans & machines alike!