Mastering Feature Engineering for Machine Learning
Updated on : 12 December, 2024, 10:00 IST
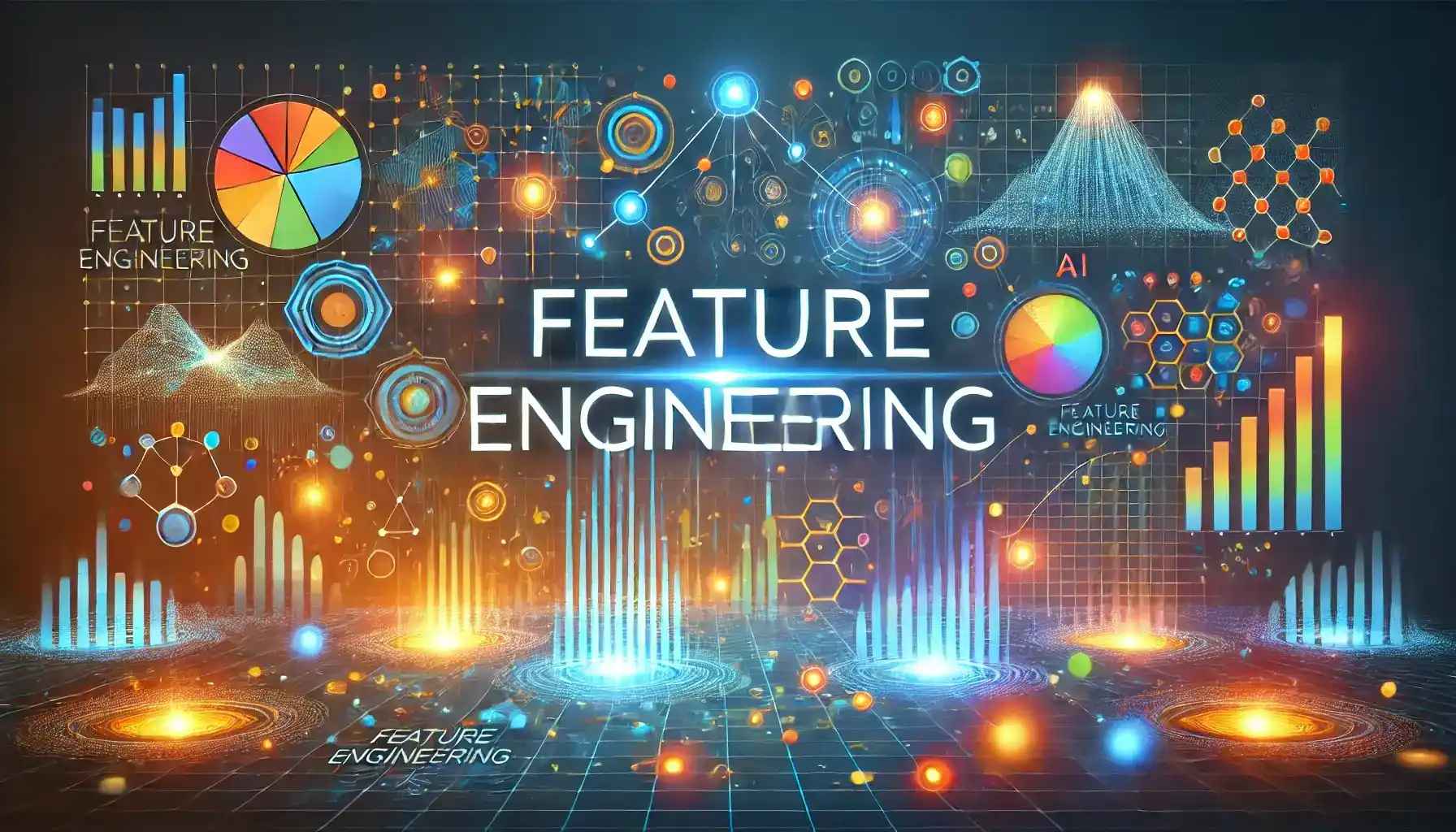
Image Source: AI-generated
Table Of Contents
- 1. What is Feature Engineering?
- 2. The Role of Feature Engineering in the Machine Learning Lifecycle
- 3. Why Feature Engineering is Crucial for Machine Learning Models
- 4. Types of Feature Engineering Techniques
- 5. Feature Engineering in Practice: Examples from Real-World Datasets
- 6. The Machine Learning Lifecycle and the Position of Feature Engineering
- 7. How AWS Supports Feature Engineering
- 8. Common Challenges in Feature Engineering
- 9. Best Practices for Effective Feature Engineering
- 10. Conclusion: The Future of Feature Engineering in Machine Learning
Table Of Contents
The Art and Science of Feature Engineering: Powering Machine Learning Success
Feature engineering is one of the most critical yet often overlooked aspects of machine learning (ML) workflows. It can significantly impact the performance of ML models, transforming raw data into meaningful features that machines can learn from. In this blog, we’ll explore the importance of feature engineering, its role in the machine learning lifecycle, and how AWS tools and services can make the process easier, faster, and more efficient.
What is Feature Engineering?
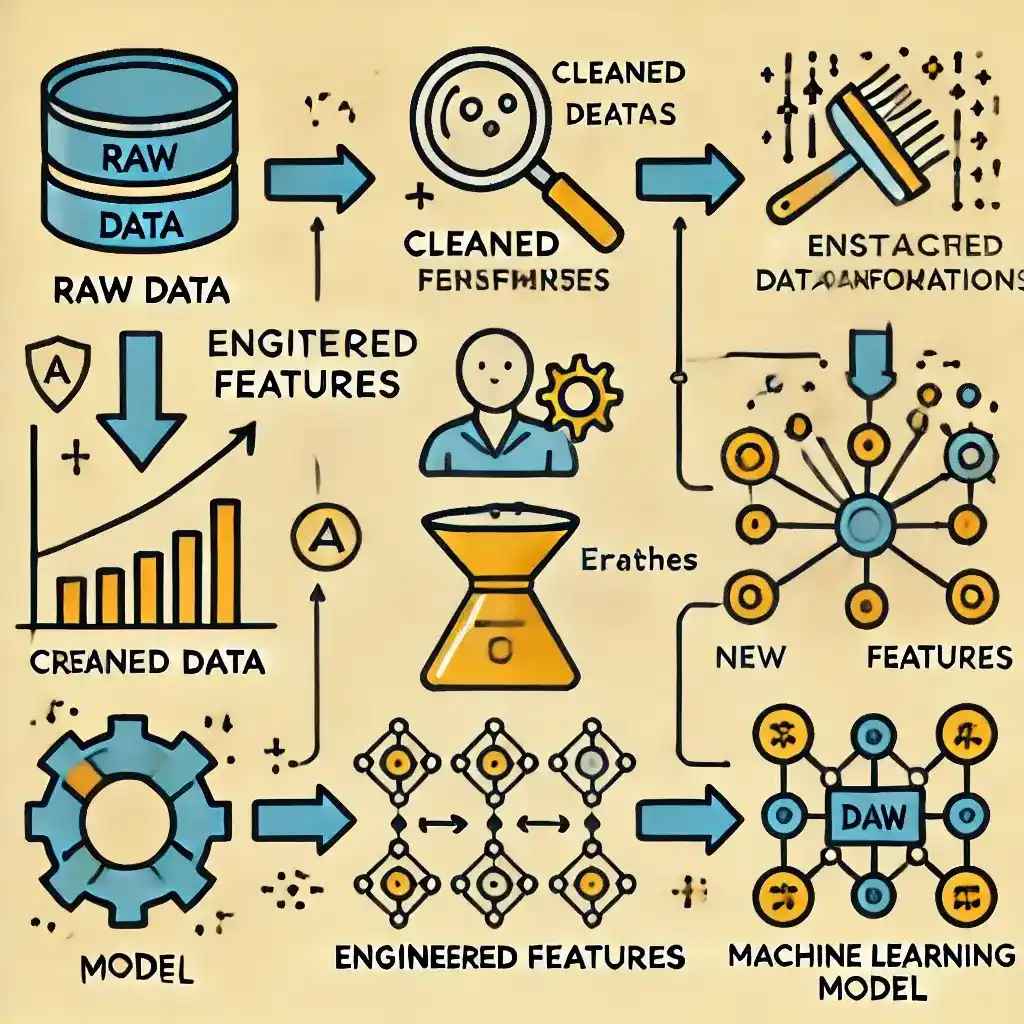
Image Source: AI-Generated
Feature engineering is the process of using domain knowledge to create, modify, or select the features that will be used by machine learning models. It is a foundational step in ML workflows, focusing on improving the predictive power of algorithms by generating better input data. This might involve handling missing data, encoding categorical variables, scaling numerical values, and even creating new features based on existing data.
The Role of Feature Engineering in the Machine Learning Lifecycle
Feature engineering plays an essential role throughout the machine learning lifecycle. Here’s a breakdown of where it fits:
- Data Collection: Gathering raw data that could become meaningful features.
- Data Preprocessing: Cleaning and transforming the raw data into features that algorithms can understand.
- Model Building: Using the engineered features to train machine learning models.
- Model Evaluation: Assessing the performance of models using validation data with appropriate features.
Feature engineering can make or break an ML model. Without it, even the most powerful algorithms may fail to make accurate predictions.
Why Feature Engineering is Crucial for Machine Learning Models
The quality of features significantly influences the success of machine learning models. Well-engineered features improve model accuracy, reduce training time, and increase the interpretability of the results. In fact, feature engineering is often the most time-consuming part of an ML project, but it yields the most significant impact on performance.
Types of Feature Engineering Techniques
Effective feature engineering can take various forms depending on the problem and data at hand. Some of the key techniques include:
Transforming Raw Data
Transforming raw data into structured and usable features is often the first step. This can include converting text into numerical features (like word embeddings or TF-IDF) or transforming timestamps into meaningful time-based features like day of the week or seasonality.
Feature Selection
Not all features are equally useful. Feature selection is the process of identifying the most relevant features, removing redundant or irrelevant ones. This helps in reducing overfitting and improving model performance.
Feature Extraction
Feature extraction involves creating new features from existing ones. For instance, combining columns or applying dimensionality reduction techniques (like PCA) to extract latent variables that represent the most critical information.
Data Normalization and Standardization
Some machine learning algorithms perform better when the data is scaled. Normalization (scaling features to a specific range) and standardization (shifting and scaling to have a mean of zero and standard deviation of one) are common techniques to prepare features for models like k-NN, SVMs, and neural networks.
Feature Engineering in Practice: Examples from Real-World Datasets
Consider a simple dataset where you want to predict house prices. Raw features like square footage
, location
, and year built
might not be sufficient. Feature engineering could include:
- Converting
year built
intoage of house
. - One-hot encoding categorical features like
neighborhood
. - Creating interaction features, such as multiplying
square footage
bynumber of rooms
to represent overall house size.
These engineered features allow ML models to better understand complex patterns in the data.
The Machine Learning Lifecycle and the Position of Feature Engineering
Data Collection
Before feature engineering, data collection is critical. Data must be gathered from different sources, be it through sensors, APIs, or databases, before any transformations can be applied.
Data Preprocessing
This is where feature engineering truly comes into play. Data preprocessing involves handling missing data, encoding categorical variables, creating new features, and preparing the data for model training.
Model Building
Once features are engineered, machine learning models are trained. The better the features, the faster and more accurate the models can be.
. Model Evaluation
After training the model, the next step is to evaluate it using test data. Well-engineered features often result in improved performance, while poor features lead to unreliable results.
How AWS Supports Feature Engineering
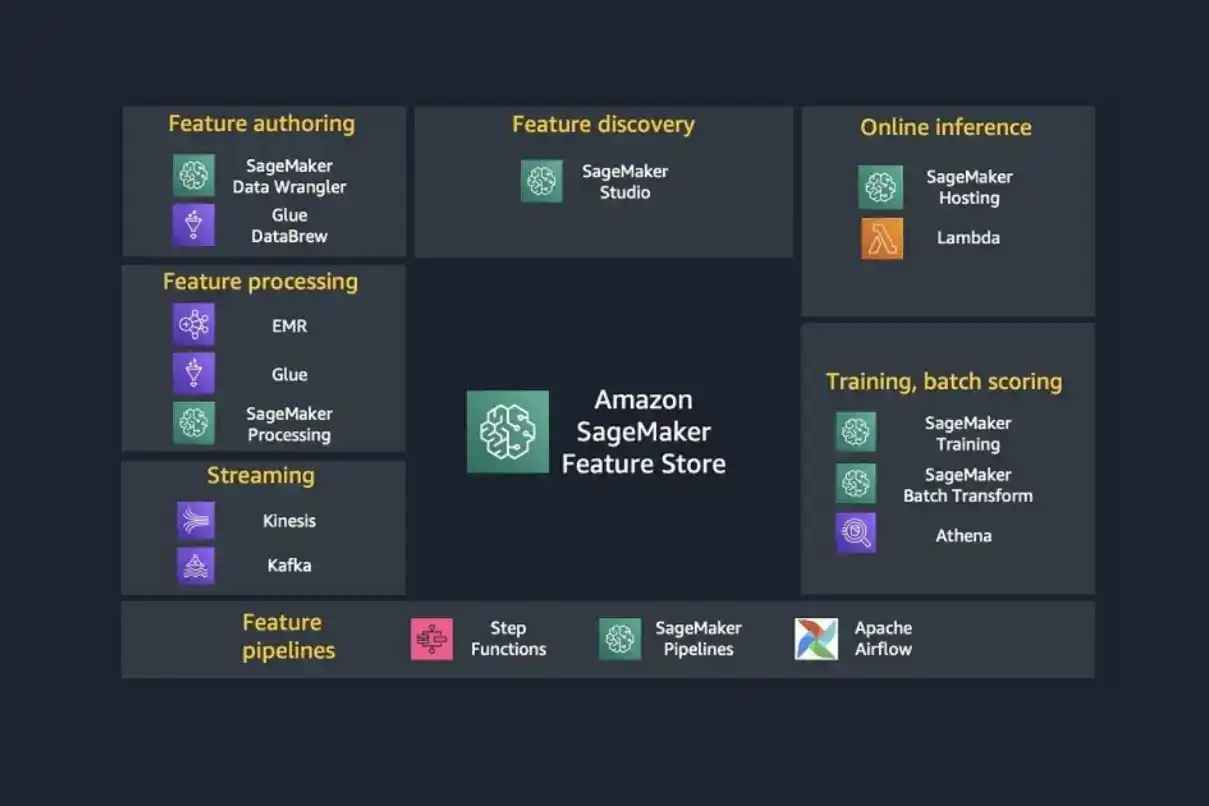
Image Source: AWS
AWS provides a suite of powerful tools to help data scientists and ML engineers with feature engineering tasks.
AWS S3 for Data Storage
Amazon S3 is a robust and scalable storage solution for storing vast amounts of data. With S3, you can securely store and access your raw datasets and engineered features, making it easy to manage data at scale.
AWS Glue for Data Transformation
AWS Glue is a fully managed ETL (Extract, Transform, Load) service that allows you to clean, enrich, and transform data. It automatically discovers and categorizes your data, helping to simplify the feature engineering process.
Amazon SageMaker for Building and Deploying Models
Amazon SageMaker simplifies the end-to-end machine learning workflow, including model building, training, and deployment. It provides built-in algorithms and tools for data preprocessing, making feature engineering an integral part of the ML model pipeline.
Common Challenges in Feature Engineering
Feature engineering is not without its challenges. Some common hurdles include:
- Dealing with Missing Data: Deciding how to handle missing values (imputation, deletion) can significantly affect model performance.
- Curbing Overfitting: With too many features, models may overfit. Feature selection and dimensionality reduction help prevent this.
- Understanding Domain Knowledge: Crafting features requires a deep understanding of the data and the problem at hand.
Best Practices for Effective Feature Engineering
To make the most out of feature engineering, consider the following best practices:
- Leverage Domain Knowledge: Use insights from the problem domain to guide feature creation.
- Use Automated Tools: AWS tools like Glue and SageMaker provide automation to speed up preprocessing.
- Iterate and Experiment: Try different features and combinations to see what works best.
- Avoid Data Leakage: Ensure that features derived from the target variable are excluded from the training data.
Conclusion: The Future of Feature Engineering in Machine Learning
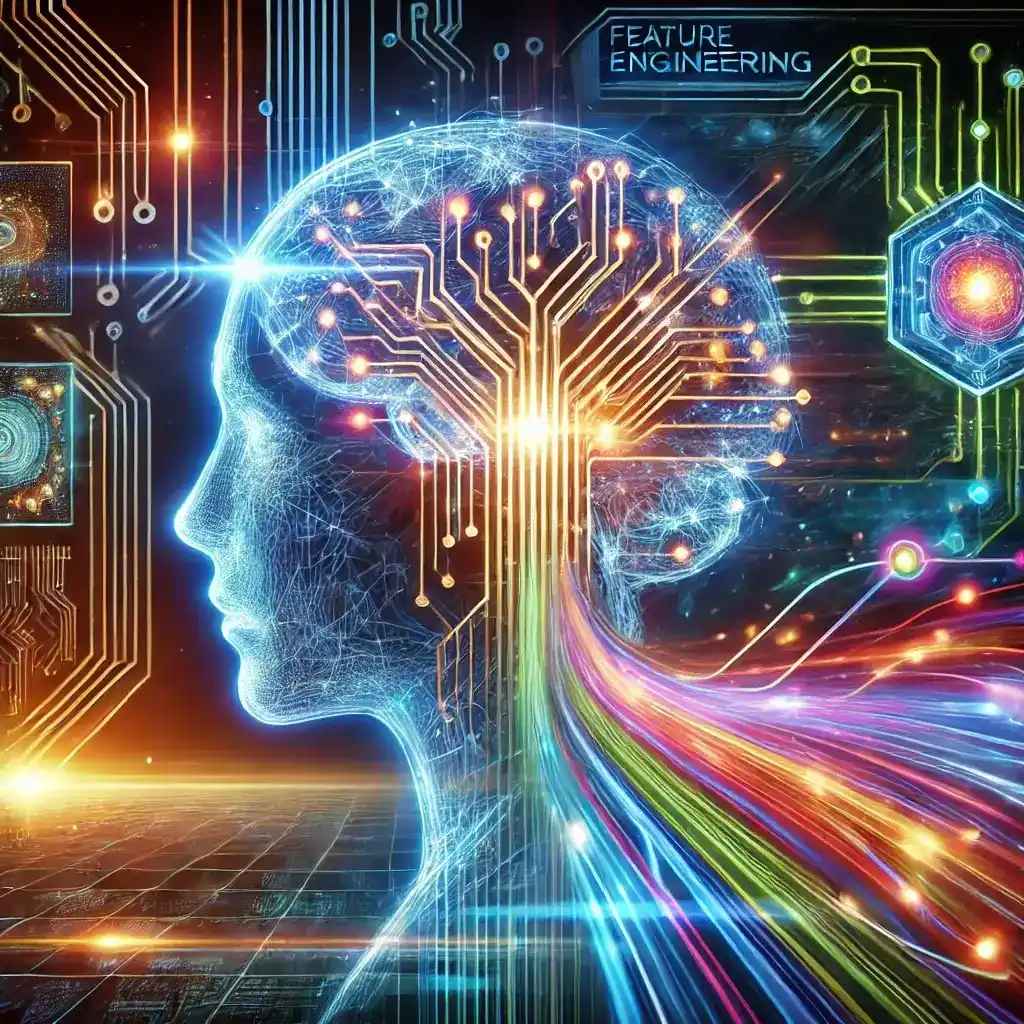
Image Source: AI-Generated
As machine learning continues to evolve, the need for effective feature engineering will only grow. With the advent of automated tools like AWS, feature engineering can be more efficient, empowering teams to unlock the full potential of their data.
In the ever-expanding world of AI, mastering feature engineering and leveraging powerful cloud-based services like AWS can drive better results, streamline ML workflows, and ensure models perform at their best.