Credit Card Fraud Detection Using Machine Learning
Updated on : 07 April 2025
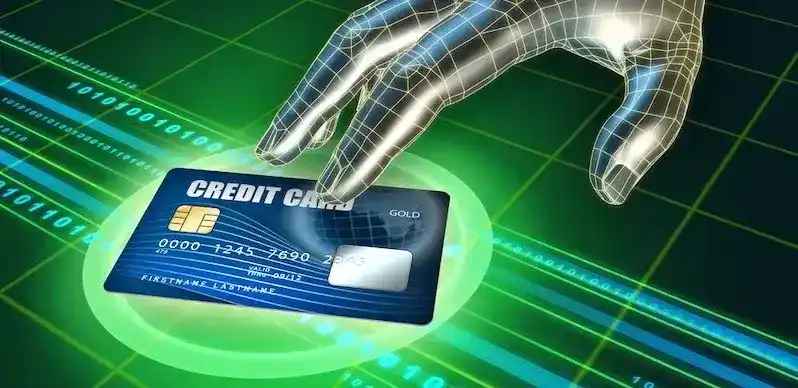
Image Source: google.com
Table Of Contents
- 1. Introduction
- 2. Common Types of Credit Card Fraud
- 3. Traditional Approaches to Fraud Detection
- 4. The Rise of Machine Learning in Fraud Detection
- 5. Real-World Industry Applications
- 6. Integrating Machine Learning into Business Models
- 7. Advanced ML Capabilities for Fraud Detection
- 8. Key Challenges in Fraud Detection
- 9. Benefits of Using Machine Learning
- 10. Business Case Study
- 11. Strategic Implementation Tips
- 12. Future Trends in Fraud Detection
- 13. FAQs
Table Of Contents
Introduction
Credit card fraud detection with machine learning is a smart way to fight financial crime. By spotting unusual spending patterns in real-time, ML models can catch fraud fast—often before it causes damage. It's a powerful blend of data, speed, and security for safer transactions.
Common Types of Credit Card Fraud
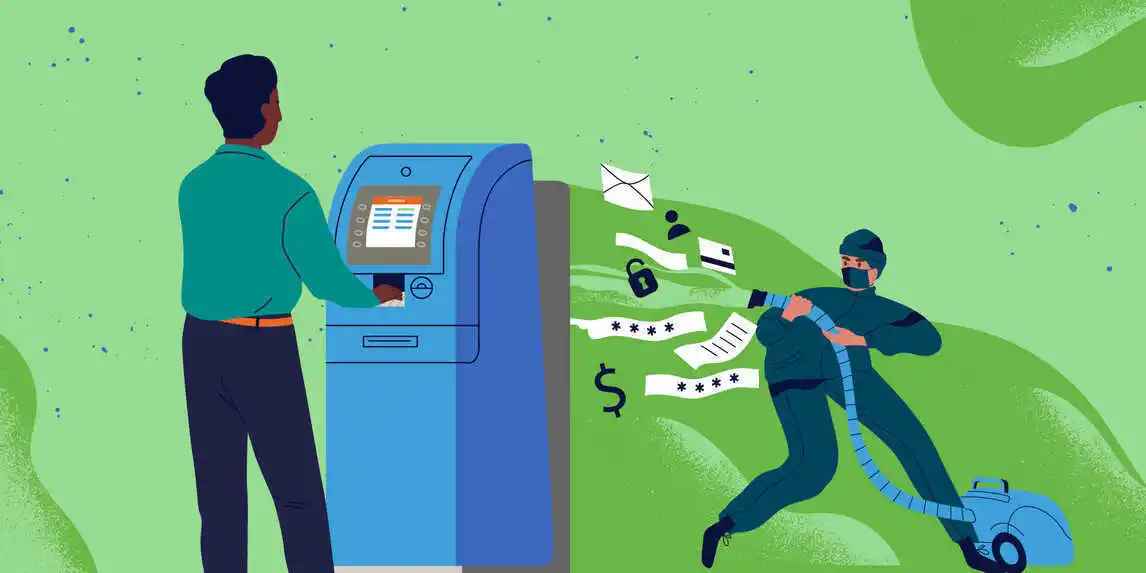
Image Source: google
-
📞 Phishing Scams
Fraudsters trick you via fake emails, calls, or texts to steal your card details by pretending to be your bank. -
👀 Skimming Devices
Tiny hidden devices at ATMs or gas pumps copy your card’s data when you swipe, without you even knowing. -
💻 Online Shopping Fraud
Your card info gets stolen during unsafe online transactions or on fake shopping websites. -
🕵️ Account Takeover
Hackers gain control of your account, change your credentials, and start making unauthorized purchases. -
🧾 Fake Credit Card Generation
Fraudsters use software to randomly generate valid card numbers and test them for working combos. -
📦 Lost or Stolen Cards
A thief finds or steals your card and quickly uses it before you report it lost. -
👤 Identity Theft
Criminals open new credit card accounts in your name using stolen personal information.
Traditional Approaches to Fraud Detection
Fraud Detection Method | Description |
---|---|
Rule-Based Systems | Use predefined rules (e.g., spending limits or location filters) to flag suspicious transactions. |
Manual Review | Human analysts inspect flagged transactions to confirm if fraud has occurred. |
Blacklists | Block transactions from known suspicious users, devices, or IP addresses. |
Transaction Limits | Set thresholds for daily or per-transaction spending to reduce large-scale fraud. |
Pattern Matching | Detect fraud by comparing current behavior to past transaction patterns. |
The Rise of Machine Learning in Fraud Detection
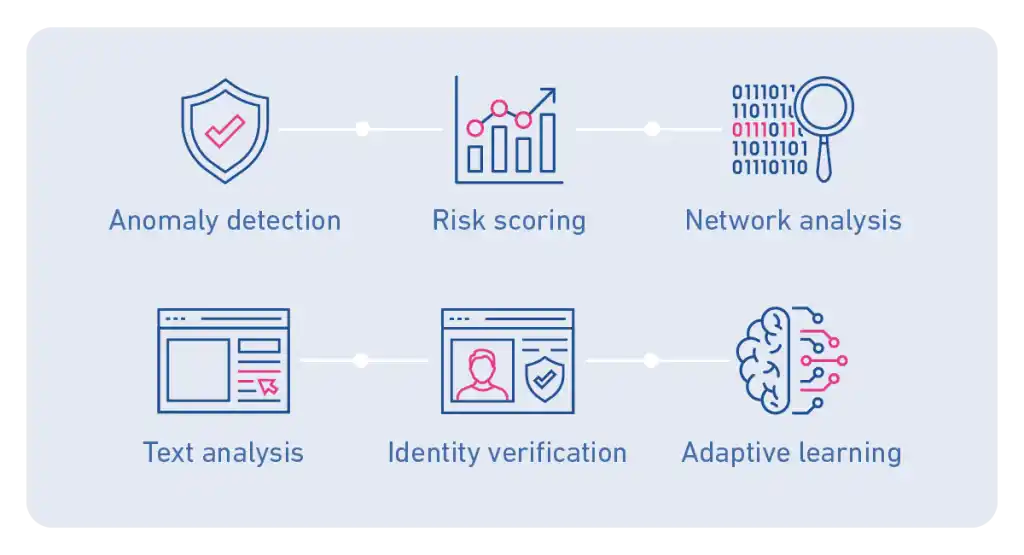
Image Source: google
-
Real-Time Detection
ML models analyze transactions instantly, flagging fraud as it happens—far faster than traditional systems. -
Adaptive Learning
Algorithms learn from new fraud patterns and evolve over time, improving accuracy without needing constant manual updates. -
Behavior Analysis
ML studies user habits (e.g., location, time, spending patterns) to detect subtle anomalies that humans might miss. -
Reduced False Positives
By understanding context, ML helps avoid flagging legitimate transactions as fraud—keeping customers happy. -
Big Data Advantage
ML can process massive datasets from multiple sources, finding fraud signals hidden in noise. -
Automation at Scale
With minimal human input, ML systems can monitor millions of transactions simultaneously and efficiently.
Real-World Industry Applications
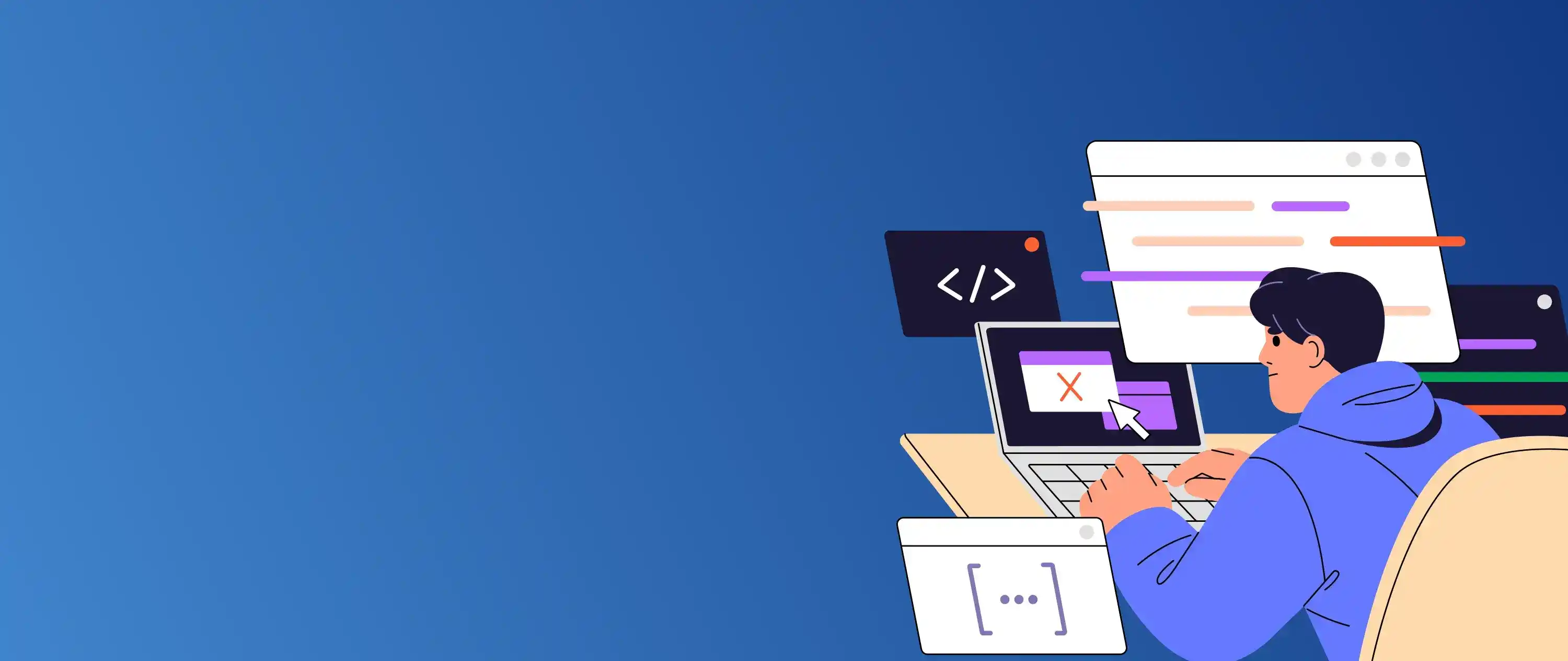
Ready to Revolutionize Your Business with Intelligent AI & ML Solutions?
🏢 Industry | 🧠 ML Fraud Detection Use |
---|---|
🏦 Banking & Finance | Detect suspicious transactions, unusual account behavior, and prevent identity theft. |
🛍️ E-Commerce | Flag fake purchases, refund scams, and card testing fraud in real time. |
📱 FinTech Apps | Analyze in-app payments, peer transfers, and automate fraud alerts. |
🏥 Healthcare | Prevent insurance fraud, fake billing, and identity misuse in patient records. |
🎮 Gaming Platforms | Stop credit card abuse in microtransactions and detect bot-driven fraud. |
Integrating Machine Learning into Business Models
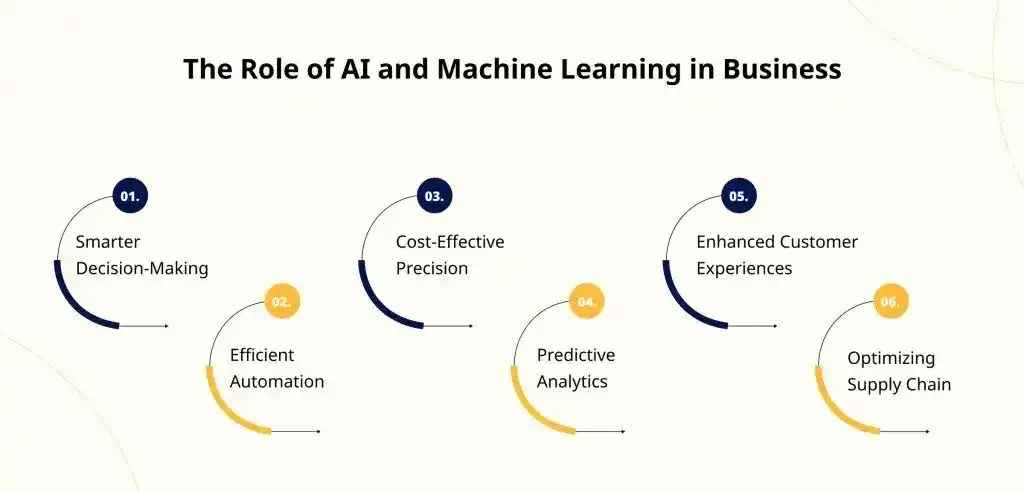
Image Source: google
-
Define the Problem
Identify specific business challenges where ML can add value—like fraud detection, customer churn, or sales forecasting. -
Collect Quality Data
Gather clean, relevant data from business operations to train accurate and reliable ML models. -
Choose the Right Model
Select algorithms that suit your needs—like decision trees for classification or neural networks for pattern recognition. -
Embed in Workflow
Integrate ML outputs into daily operations, such as flagging risky transactions or automating decisions. -
Monitor & Improve
Continuously track performance, retrain models with new data, and refine them for better accuracy over time. -
Ensure Compliance & Ethics
Maintain transparency, privacy, and fairness while deploying ML in customer-facing or sensitive areas.
Transform Your Business with Hexadecimal’s Blockchain Expertise
Advanced ML Capabilities for Fraud Detection
ML Capability | Description |
---|---|
Anomaly Detection | Identifies unusual transaction patterns that deviate from normal user behavior. |
Supervised Learning | Trains models on labeled fraud data to classify future transactions as legitimate or fraudulent. |
Unsupervised Learning | Detects hidden fraud patterns without needing labeled data, useful for unknown fraud types. |
Reinforcement Learning | Learns and adapts from feedback in real-time to improve detection over time. |
Natural Language Processing (NLP) | Analyzes textual data like user reviews or support chats to detect potential fraud signals. |
Key Challenges in Fraud Detection
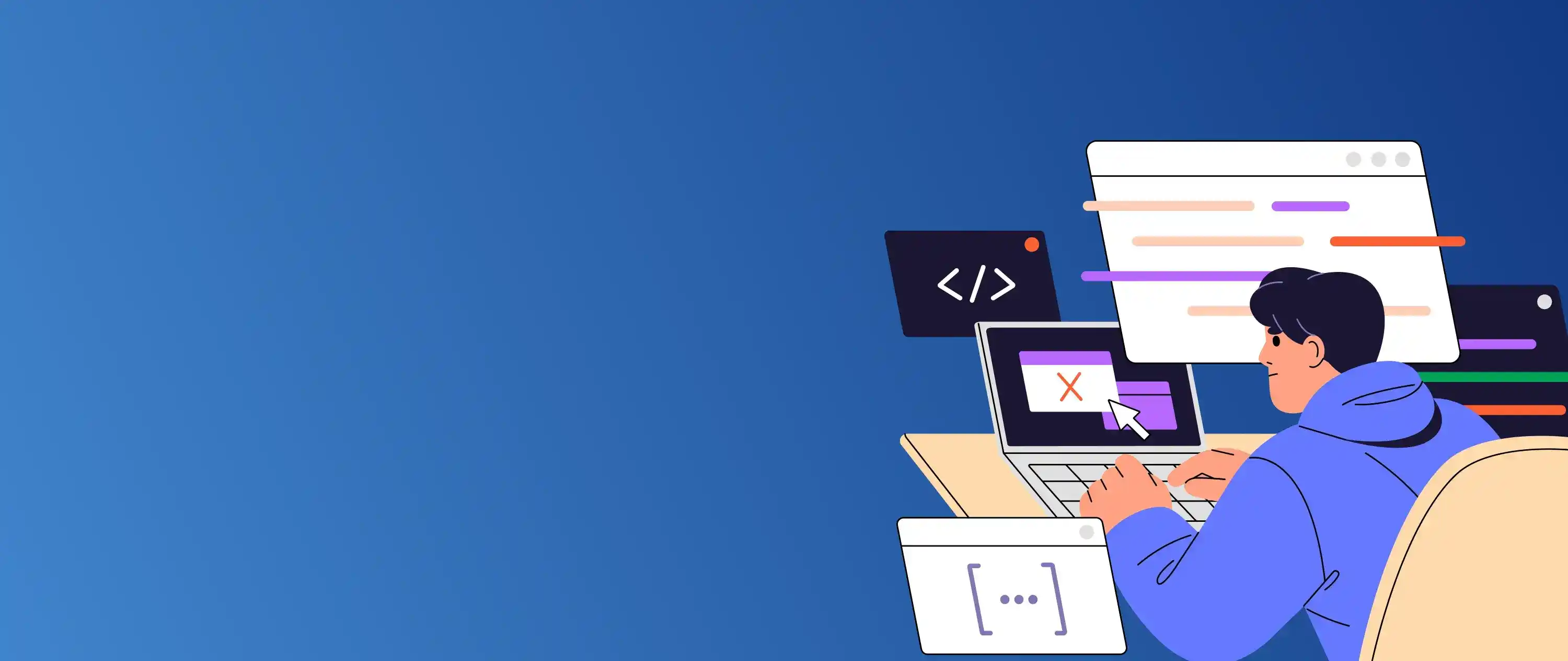
Want to Elevate Your Financial Security with Blockchain-Powered Fraud Detection?
-
🎭 Evolving Fraud Tactics
Fraudsters keep changing strategies, making it hard for static systems to stay effective. -
🚫 High False Positives
Legitimate transactions often get flagged, causing inconvenience and loss of customer trust. -
⚖️ Data Imbalance
Fraud cases are rare compared to normal ones, which can bias machine learning models. -
⏱️ Real-Time Detection Needs
Systems must detect fraud instantly without slowing down user experience or transactions. -
🛡️ Privacy & Compliance
Solutions must follow strict data protection laws (like GDPR), which adds complexity to system design. -
🔄 Continuous Learning Requirement
Fraud detection models need regular updates and retraining to stay accurate and relevant.
Benefits of Using Machine Learning
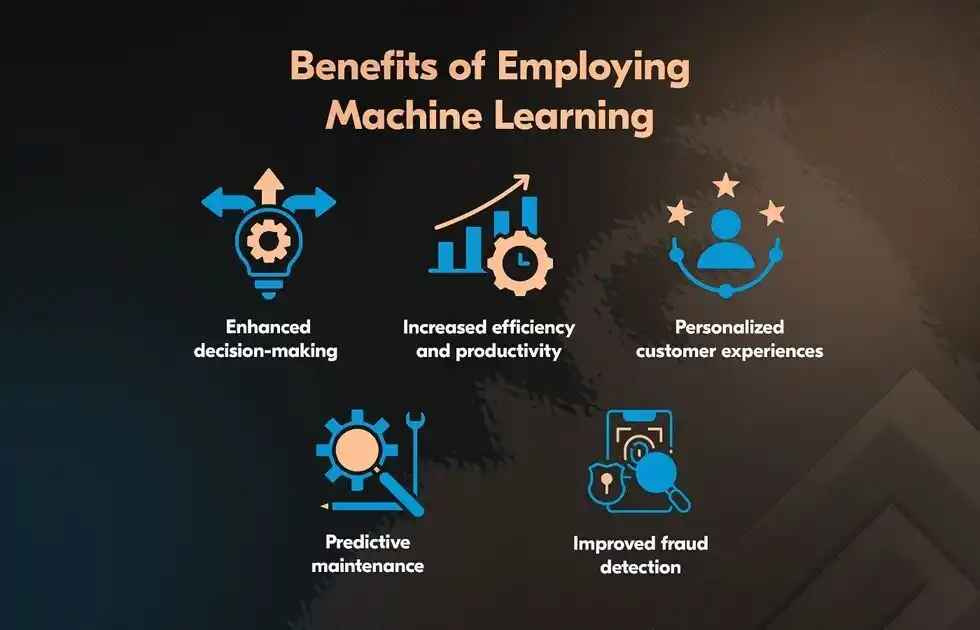
Image Source: google
✅ Benefit | 📌 Description |
---|---|
Real-Time Detection ⚡ | Instantly flags suspicious transactions, reducing response time and damage. |
Improved Accuracy 🎯 | Reduces false positives and better detects complex fraud patterns. |
Scalability 📈 | Handles millions of transactions efficiently without human intervention. |
Adaptive Learning 🔁 | Continuously improves by learning from new data and emerging fraud tactics. |
Cost Efficiency 💰 | Minimizes manual work, reducing operational costs and boosting ROI. |
Enhanced User Trust 🤝 | Accurate detection builds customer confidence in digital transactions. |
Business Case Study
-
About
A leading mobile app development company delivering innovative digital solutions across industries. -
Challenge
Clients in fintech and e-commerce needed robust, real-time fraud detection systems integrated into their apps. -
ML-Powered Solution
Hexadecimal's implemented machine learning models to detect and prevent fraud using user behavior analytics and anomaly detection. -
Key Technologies
Used algorithms like decision trees, logistic regression, and neural networks along with real-time data pipelines. -
Impact
- 60% reduction in fraudulent transactions
- Improved customer trust
- Seamless integration without affecting app performance
- Industry Reach
Delivered ML-based fraud solutions for global clients across fintech, retail, and healthcare domains.
Strategic Implementation Tips
Strategy Tip | Description |
---|---|
Start Small & Scale | Begin with a pilot project to test ML models, then expand based on results. |
Use Quality Data | Ensure clean, relevant, and diverse datasets to train accurate models. |
Cross-Functional Teams | Involve data scientists, domain experts, and developers for effective implementation. |
Monitor & Improve | Continuously evaluate model performance and retrain with new data. |
Ensure Compliance | Align ML systems with privacy laws (like GDPR) and ethical standards. |
Integrate with Systems | Embed ML into existing workflows and tools for smooth operations. |
Future Trends in Fraud Detection
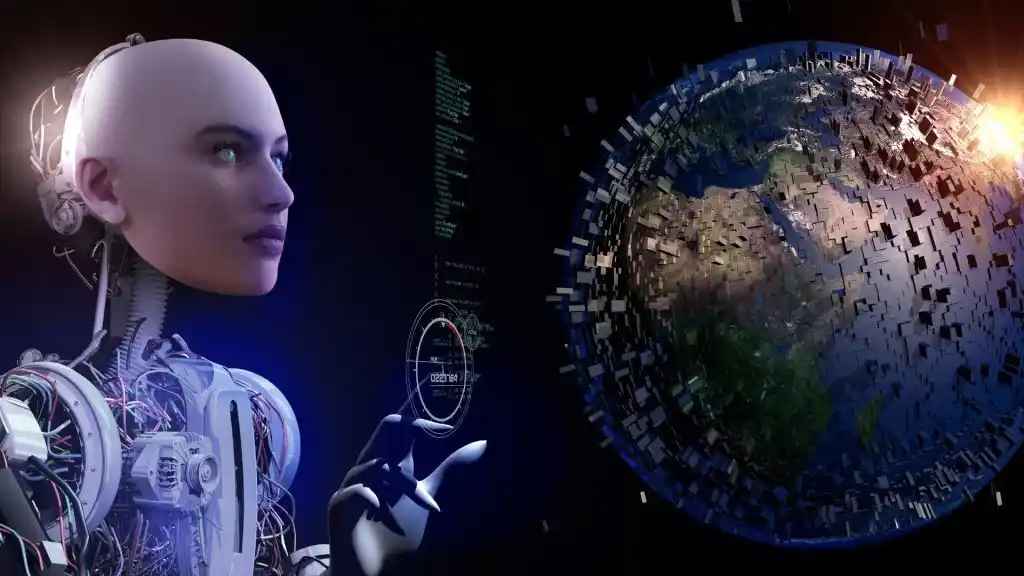
Image Source: google
-
🧠 AI-Powered Automation
Advanced AI models will automate fraud detection end-to-end, from analysis to action—reducing human involvement. -
🌐 Real-Time Global Monitoring
Cross-border fraud detection systems will enable faster identification of international fraud patterns. -
🔗 Blockchain Integration
Transparent and tamper-proof blockchain systems will help verify transactions and identities more securely. -
📱 Biometric Authentication
Fingerprint, facial recognition, and voice-based verification will add extra layers of fraud prevention. -
🎯 Hyper-Personalized Detection
Systems will tailor fraud detection to individual user behavior, improving accuracy and reducing false positives. -
🧩 Federated Learning
ML models will be trained across multiple decentralized sources without compromising user privacy. -
🚨 Predictive Analytics
Using historical and behavioral data, systems will not just detect but predict potential fraud before it happens.
FAQs
Q.1.What is credit card fraud detection using machine learning?
A : It’s the use of ML algorithms to analyze transaction data and identify suspicious or fraudulent activity automatically.
Q.2.How does machine learning detect fraud?
A : ML models learn from past transaction patterns (fraudulent and legitimate) to spot unusual behavior in real-time.
Q.3.What types of algorithms are used?
A : Common algorithms include decision trees, logistic regression, random forests, neural networks, and k-nearest neighbors (KNN).
Q.4.What kind of data is used for training ML models?
A : Transaction data like amount, location, time, device info, and user behavior (e.g., spending habits).
Q.5.Why is machine learning better than rule-based systems?
A : ML adapts over time, handles complex patterns, and reduces false positives—unlike static, predefined rules.
Q.6.Can ML detect fraud in real time?
A : Yes! Many systems are designed to flag suspicious activity within milliseconds of a transaction occurring.